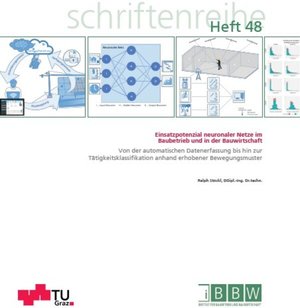
Einsatzpotenzial neuronaler Netze im Baubetrieb und in der Bauwirtschaft
Von der automatischen Datenerfassung bis hin zur Tätigkeitsklassifikation anhand erhobener Bewegungsmuster
von Ralph StöcklWir begegnen künstlicher Intelligenz nahezu täglich. Sei es bei selbstfahrenden Autos, Gesichts-, Bild- oder Spracherkennung, personalisierter Werbung, individuell abgestimmten Musikvorschlägen, Smart Homes, Wetter- und Sportberichten oder beim Einkaufen. Die hierbei eingesetzten neuronalen Netze ermöglichen auf Basis effizienter Datenanalysen das Erstellen von effektiven Vorhersagen, welche den menschlichen Alltag wesentlich erleichtern. Aus diesem Grund setzen mittlerweile zahlreiche Branchen wie beispielsweise die Medizin, der Verkauf oder die Automobilindustrie auf künstliche Intelligenz, um Arbeitsprozesse zu verbessern. Der Baubetrieb und die Bauwirtschaft greifen bisher noch vergleichsweise wenig auf die Potenziale neuronaler Netze zurück. Um den Weg der künstlichen Intelligenz auf die Baustelle zu ebnen, verfolgt die vorliegende Arbeit das Ziel, einen ganzheitlichen Ansatz für die Anwendung neuronaler Netze aufzuzeigen, welcher alle notwendigen Schritte von der Datenerhebung bis hin zur Modellbildung beinhaltet. Hierfür werden zunächst die theoretischen Grundlagen erläutert und anhand ausgewählter Prognosebeispiele, welche die Wichtigkeit der Verteilungsanalyse von Trainings- und Testdaten hervorheben, vertieft. Anschließend stehen sowohl bauferne als auch baubetriebliche und bauwirtschaftliche Einsatzmöglichkeiten im Fokus der Betrachtung, wobei die Inputparameterauswahl für Vorhersagen des Gesamt-Aufwandswertes und der Produktivitätsverluste eine tiefergehende Betrachtung erfährt. Basierend auf einer umfassend durchgeführten Literaturrecherche wird gezeigt, dass ein wesentlicher Grund, weshalb künstliche Intelligenz bei Kosten-, Dauer- oder Produktivitätsprognosen bisher keine breiteWe use artificial intelligence almost every day. Be it in self-driving cars, face, image, or voice recognition, personalised advertising, music suggestions, smart homes, weather, and sports reports, or shopping. These neural networks enable effective predictions based on efficient data analyses, which make human life much easier. For this reason, numerous industries, such as medicine, sales, or the automotive industry, are now relying on artificial intelligence to improve their work processes. However, the construction industry has made comparatively little use of the potential of neural networks yet. To pave the way for artificial intelligence on the construction site, this PhD thesis aims to present a holistic approach for the application of neural networks, which includes all necessary steps from data collection to model building. For this purpose, first, the theoretical background is explained and deepened through selected forecast examples, which emphasise the importance of the distribution analysis of training and test data. Subsequently, the focus is on use cases in construction management and economics, whereby the input parameter selection for predictions of the labour consumption rate and the productivity losses is examined in greater depth. In the next step, the extensive literature review has shown that one major reason why artificial intelligence is not widely used in cost, duration, or productivity forecasting is data availability. The used data sets often have few cases. Thus they are not suitable for being analysed by artificial intelligence. For this reason, five concepts for the automatic collection of working hours with simultaneous area and activity allocation are developed. Subsequently, one of these concepts is selected and evaluated during reinforced concrete work on a construction site. The result is that global navigation satellite systems in combination with beacons based on Bluetooth Low Energy are very well suited for this task because they enable reliable data collection inside and outside the building. Based on the collected data, the procedure for using neural networks is then illustrated. This example pursues the goal of recognising formwork, reinforcement, and concreting activities based on the workers' movement patterns. During the iterative modelling process, it turns out that neural networks have great potential in the classification of selected activities based on duration, distance, number of ways, and warehouse visits as well as average and maximum speed.